Exploratory Data Analysis (EDA) helps single out potential observations from the data which can then be used to make decisions. It acts as a foolproof mechanism for businesses. EDA is essential for any successful data analytics project. The EDA is crucially significant across the Types of Data Analytics. It is a central area in which the Data Analytics Certification holders conduct the impactful analysis of data.
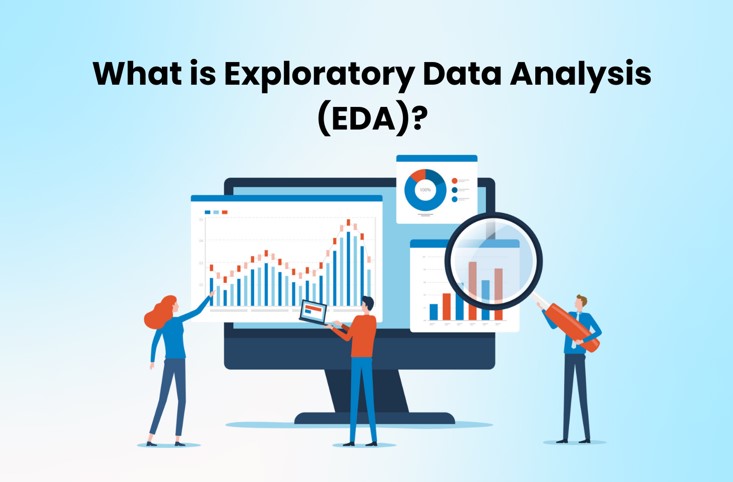
Table of Contents
- What is Exploratory Data Analysis?
- The Role of EDA in Data Analytics
- Data Analytics Certification and EDA
- The Core Components of Exploratory Data Analysis
- Descriptive Statistics
- Data Visualisation
- Outlier Detection
- Correlation Analysis
- The Nexus of EDA and Types of Data Analytics
- Descriptive Analytics
- Diagnostic Analytics
- Predictive Analytics
- Prescriptive Analytics
- Conclusion
What is Exploratory Data Analysis?
We’ll now decode the basics of exploratory data analysis, and get ready to embark on our new journey. EDA is a particular process during which data analysts necessarily inspect the unprocessed data sets and make out the major patterns and insights. EDA is like uncovering what is beneath the surface like an onion making a distinction between the simple and the complex, unlike high-level algorithms getting right to the difficult stuff.
EDA’s significance as a foundational ability is frequently emphasised in data analytics certification programs. It gives experts the skills to sift through enormous information and turn it from raw data into engaging stories that can influence wise decisions.
The Role of EDA in Data Analytics
Since EDA is the cornerstone of data analytics, it is essential in determining how the analytical process is carried out. It would be similar to having raw data without the insights obtained from EDA or having a treasure map without knowing its symbols. This stage acts as a litmus test for the accuracy and dependability of the available data, in addition to aiding in identifying trends and patterns.
It is imperative for anyone hoping to advance their career and obtain a data analytics certification to learn EDA. It is the starting point for a thorough understanding of the data landscape and offers the abilities required to convert data into insight that can be put to use.
Data Analytics Certification and EDA
Acquiring a certification in data analytics requires learning about several aspects of the profession. In this dance, EDA steals the show as it leads experts through the complexities of real-world datasets. It’s a crucial factor that distinguishes skilled analysts from inexperienced ones; it’s more than just a checkbox in the learning process.
Knowing the subtleties of EDA improves your analytical skills in certifications. Ensuring that you can criticalaly assess both organised and unorganised data is a necessity. Certification in data analytics that includes EDA as one of the main modules, ensures that data professionals are well equipped with knowledge and skills to cater to the emerging analytical needs of the World as it turns into a Data chaotic world.
The Core Components of Exploratory Data Analysis
Descriptive Statistics
Descriptive statistics are the first step in the EDA process. These statistical measurements give an overview of the data’s shape, dispersion, and central tendencies. In the first few chapters of our investigation, the variables mean, median, mode, and standard deviation are the main characters.
Data Visualisation
Data visualisation is one of EDA’s most powerful capabilities. Visual representations, such as scatter plots and histograms, give raw data life and make trends and patterns stand out. The revolutionary potential of visualisation in EDA is acknowledged by a data analytics certification that is worth its weight.
Outlier Detection
The rebels in the data universe, known as outliers, have a big influence on the narrative analysis. EDA is the painstaking process of locating and interpreting these anomalies: are they mistakes, or do they disclose a hidden aspect of the data?
Correlation Analysis
Correlation analysis is used for variables. It reveals the relationships between several data points, offering insightful information about how adjustments to one variable may have an impact on another.
The Nexus of EDA and Types of Data Analytics
Descriptive Analytics
Among data analytics techniques, descriptive analytics is based on the understanding gained from exploratory data analysis. Proficiency in summarising, interpreting, and presenting data is essential for individuals working in this core area of analytics.
Diagnostic Analytics
EDA is the analytics equivalent of Sherlock Holmes, delving deeper into diagnostics and helping to comprehend the ‘why’ underlying trends and anomalies pave the way for making well-informed decisions.
Predictive Analytics
EDA leads the path as we navigate the realm of predictive analytics. It gives analysts the ability to forecast future events with accuracy by seeing patterns and trends, which is a highly desired skill in the fast-paced field of data science.
Prescriptive Analytics
Prescriptive analytics in the field of analytics feeds on judgments and suggestions. As the cornerstone, EDA makes sure that these suggestions are based on a thorough comprehension of the nuances of the data rather than being arbitrary guesses.
Conclusion
Exploratory Data Analysis is the nameless hero of data analytics. It guides experts through the complex layers of information by transforming unprocessed data into a story. To individuals who want to become certified in data analytics and further their career, becoming an expert in EDA is like having a map in a foreign land. Remember that this is a mindset, not just a phase, as we wrap up our exploration of EDA. Analytical thinking aside, the capacity to analyse, evaluate, and comprehend facts is a very valuable quality. Thus, seize the opportunity, arm yourself with EDA’s might, and let the data reveal its mysteries to you. You will become a true explorer in data analytics.
Leave a Reply